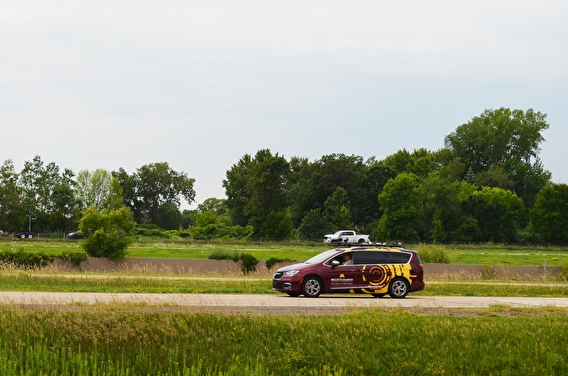
Partially autonomous vehicles (AVs) are already on Minnesota roads, and fully autonomous ones may not be far behind. As these vehicles evolve, transportation agencies have practical questions about accommodating them. For example, AVs rely on visible lane markings for navigation, but what happens if lines are partially covered by snow or hidden in work zones? Will winter road maintenance or work-zone practices need to change?
To gain insight, U of M researchers used the University’s MnCAV automated vehicle for a series of experiments on highways and local roads. The work was led by Professor Rajesh Rajamani of the Department of Mechanical Engineering and funded by the Minnesota Local Road Research Board and the Minnesota Department of Transportation (MnDOT).
“Local transportation agencies and MnDOT need to better understand the implications of autonomous vehicles on the design, maintenance, and operations of our roads,” says Victor Lund, traffic engineer with St. Louis County Public Works and the project’s technical liaison. “The results of this project will shape our ongoing inquiry as technology progresses.”
The MnCAV vehicle, part of the University’s MnCAV Ecosystem, is a 2021 Chrysler Pacifica minivan that serves as a customizable, experimental testbed for CAV research. It can navigate highways and traffic without a human driver’s assistance—including handling curves and lane changes at highway speeds.
For this study, researchers evaluated the vehicle at the MnROAD testing facility to understand its performance in ideal conditions and ensure it was working correctly. Next, the AV was driven on local freeways with varying snow cover to assess its ability to find lateral position and stay within lane boundaries. (Investigators manually drove the vehicle for safety when lane markings were covered.)
“Today’s AVs tend to function best in ideal conditions, and most industry testing takes place in sunny weather locations,” Rajamani says. “Less is known about how well they’re prepared for the challenges of winter driving, work zones, and heavy traffic.”
To better understand AV impact on traffic flow and operations, the vehicle was driven in light, medium, and heavy traffic on local highways to compare differences in speed, acceleration, and following distance between its manual and autonomous driving modes.
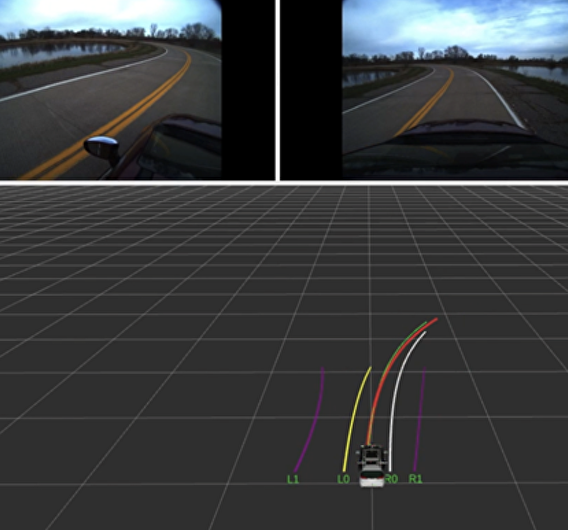
Finally, the vehicle’s performance in work zones was tested at the MnROAD facility, where it was driven in autonomous mode through a variety of work-zone configurations including temporary and conflicting lane markings and cones or barrels placed at various spacings
Through this research, the project team was able to identify implications and further questions for winter road maintenance, work zones, and traffic flow in relation to autonomous vehicles.
Initial tests showed the experimental AV had excellent steering on straight roads but had offset errors on curves; also, it was good at matching a lead vehicle’s speed at a steady state but cut-ins from other vehicles caused lurching. The research team made improvements to the adaptive cruise control system on the vehicle to enable it to smoothly handle heavy traffic.
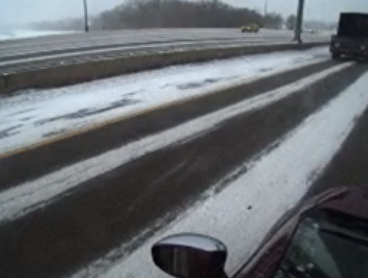
Snowy conditions and work zones presented a challenge for the AV. When snow partially or fully impeded lane line visibility, it significantly reduced lane detection. This would make autonomous control very challenging even with light snow cover, Rajamani notes.
In work zones, cones that didn’t impede lane markings didn’t impact detection, but cones placed on top of markings needed to be at least six feet apart; barrels reduced detection slightly more than cones. In autonomous mode, the AV couldn’t detect temporary lane markings or operate with conflicting markings.
The AV showed promise, however, for creating smoother traffic flows in varying traffic conditions. In light and medium traffic, it accelerated and decelerated more gradually than the preceding vehicle, indicating that AVs can decrease speed variability and improve traffic flow. In heavy traffic, despite stop-and-go conditions, the AV consistently performed more smoothly than all the manually driven vehicles it was following.
These findings reveal possible strategies that transportation agencies could use to make roads more AV-friendly. For example, winter maintenance crews could clear snow from lane lines, and agencies may reconsider how much snow is acceptable on their less-used roads. Early warning of upcoming construction zones could allow AV drivers to transition to manual driving mode. The potential benefits of AVs for traffic operations—such as more vehicle capacity—could reshape agencies’ infrastructure planning.
“And,” Rajamani adds, “this research could help vehicle manufacturers understand regional considerations for AVs—Minnesota winters included.”
—Megan Tsai, contributing writer